Machine Learning & AI
With a high volume of rich, consistent, and accessible video data captured by the full scope of our AIVR systems, integrated Machine Learning automatically finds what you need to see the most.
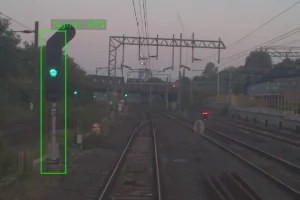
Accessing data in the AIVR platform can be enhanced through the use of automatic asset detection features, developed by our in-house Machine Learning team. We work closely with rail industry experts and technology partners to assure our datasets provide vital insight to assets and componentry.
Get In TouchHere are some examples of AI in AIVR:
Thermal Hotspots
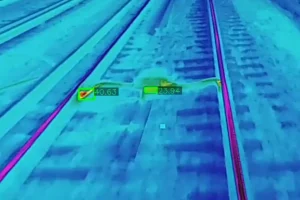
Thermal footage is recorded alongside Forward-Facing Video, where the readings and images are viewable concurrently in the AIVR platform. Machine Learning models automatically pinpoint frames within the footage which exceed a pre-set temperature, allowing users to review both FFV and Thermal data to identify whether intervention is required.
Track Componentry
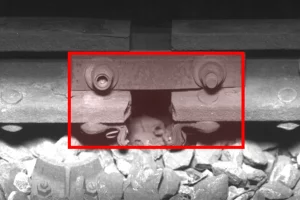
Line-scanning data captured by the AIVR Focus system provides an incredibly comprehensive capture of the track using underbody cameras. Machine Learning is applied to the high-definition imagery to automatically detect componentry such us Fish Plates, Fasteners, Switches and Crossings, Welds, and further points of interest. As further data enters the system the conditions of these assets can be assessed and highlighted as an ‘issue’ for further investigation.
Graffiti
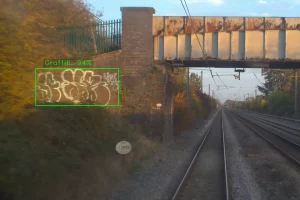
Thousands of images of lineside graffiti were annotated in rapid time to create a dataset to train a Machine Learning model that detects graffiti of all different types and locates them on the rail map. This automated detection enables targeted and effective response to areas of vandalism and trespass.
Signals
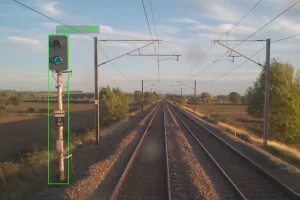
Using repetitive data capture from any AIVR device in any location, signals and their ID plates are being detected and mapped. Every time each signal is detected, the location details are verified and confirmed to build a dynamic asset map, enabling engineers to navigate to any signal by its unique ID. Similar AI is being applied to detect and map OLE structure IDs.
Scrap Rail
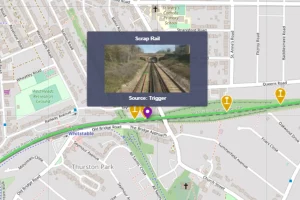
Scrap Rail, as well as other lineside items such as ballast bags, are being detected using AI. As part of the continuous work on Track Worker Safety, hazards in the cess and four foot are identified and located on the rail map. This continuously evolving AI helps create a safer working environment and the ability to locate, remove or redeploy assets such as scrap rail.
Corona Discharge
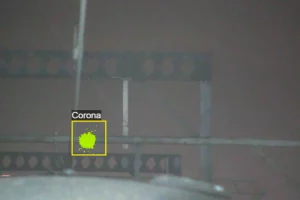
UV Corona cameras which are installed onto a measurement fleet or in-service train enable automated data capture with real-time data transmission; in order to capture the electricity OLE. Using Machine Learning models which identify occurrences of Corona within the UV data, refined processes can be developed to monitor OLE performance and support predictive maintenance.
Balance Weights
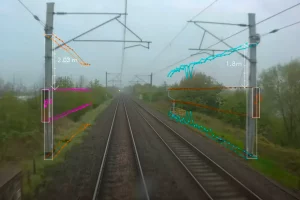
As part of wider OLE maintenance practices, we have used AIVR Machine Learning to automatically identify and measure balance weights along the trackside to support predictive monitoring of Overhead Wires.